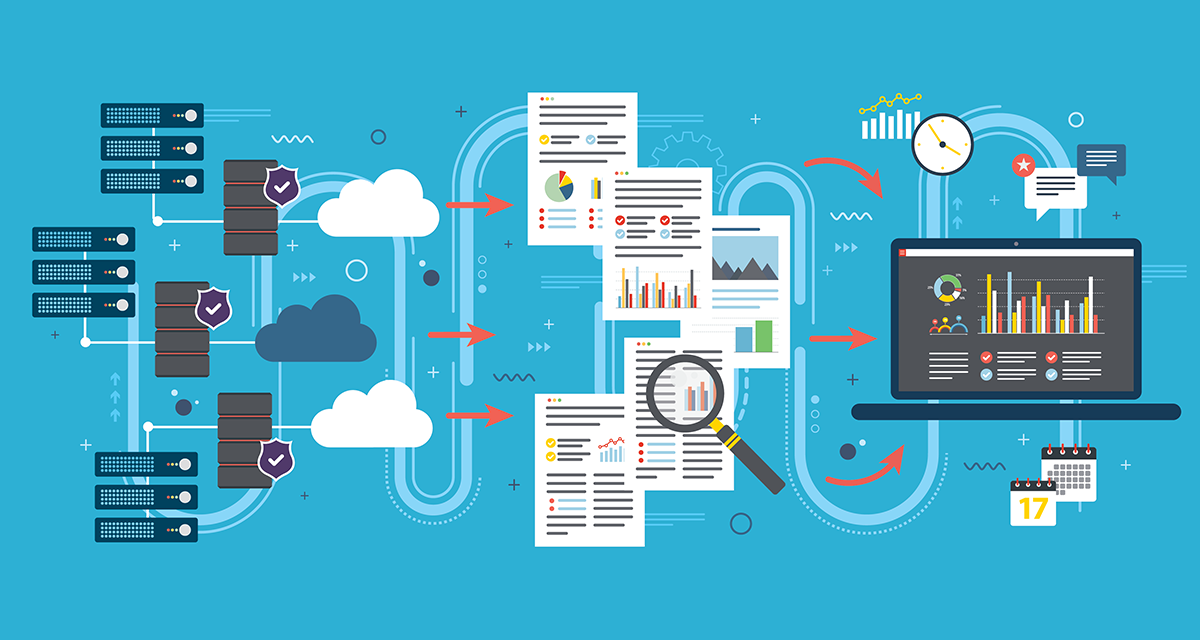
How Synthetic Data Fuels Digital Transformation in Healthcare
The healthcare sector is on the verge of a revolution in data and analytics, but the advancement of data-driven decision making has been hampered by difficulties in updating legacy systems, as well as challenges stemming from disparate data sources. With the growing push to digitize patient and claims information, the evolution of healthcare data exchange standards has come a long way to address some of these problems, but not all of them.
Shortly after the 2019 release of the updated Fast Healthcare Interoperability Resource (FHIR) standard, it became the default for health IT certification, and it rapidly gained support from stakeholders throughout the sector. FHIR R4 added support for more data formats, including JSON and XML. Although industry support for FHIR has grown rapidly, the healthcare sector continues to face IT modernization challenges.
Difficulties in Achieving Digital Transformation for Healthcare
The healthcare technology landscape will get even more complicated as more connected devices enter the fray. As the internet-of-medical-things trend takes shape, the variety and volume of data that must be ingested by modern electronic health record (EHR) systems will grow exponentially, as will the number of different devices and systems that must be rigorously tested.
The question is: Are healthcare software systems and analytics tools ready for the deluge of data and web-based health applications?
For organizations using traditional methods for software testing, the process of modernization involves a significant amount of manual effort preparing and managing test data. When advanced technology like AI interacts with these systems, the same data quality problems appear, and this can lead to biased models that aren’t able to achieve results.
For example, training a machine learning algorithm on a limited set of patient data will severely limit its ability to diagnose illness in real-world scenarios, whereas training on larger data sets can be difficult due to privacy and security concerns. In fact, the lack of high-quality and high-volume data is one of the key factors leading to the failure of AI-driven platforms like Google Health and IBM Watson’s Health Analytics.
Electronic Health Care Record (EHR) modernization has been a focal point for healthcare for years, and FHIR serves to push the envelope on interoperability further by providing software developers with implementation tools and the support of a global community. Data exchange standards like HL7 have helped providers and insurers share information to some degree, but some major roadblocks persist when it comes to provisioning data for testing:
- Many disconnected legacy systems and data siloes make modernization time consuming and expensive
- Information privacy and security become even more prominent concerns when patient and cost data is centralized.
- Limitations in how patient data can be shared and used limit the kinds of software and analytical tools that can draw on live data
- Production data is frequently inconsistent or incomplete, making it difficult for testers to account for every possible scenario.
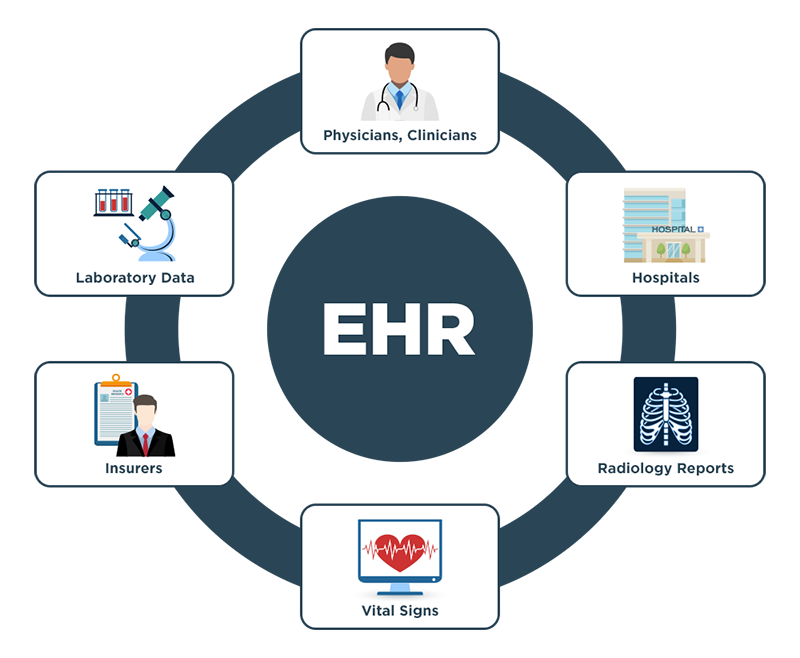
Problems ranging from data entry mistakes to differences in international and U.S.-based medical coding standards have made it difficult to leverage advanced analytics and AI tools, since these technologies rely on robust, large data sets to train and refine. Because of differences in medical coding standards like ICD-10 and international data standards like SNOMED, there also inconsistencies in how data is entered in EHR systems to begin with, and a lot of the data that would be useful for analysis exists in unstructured formats, across disparate notes and spreadsheets.
The volume and variety of data entering healthcare IT ecosystems is also set to expand with new regulatory mandates such as the Interoperability and Patient Access rule, which mandates that healthcare organizations make health information easily accessible to patients. Along with FHIR, this change is pushing providers and insurers toward the adoption of APIs, a healthcare technology market that is expected to grow at a 6.3% CAGR through 2027. The largest share of this growth can be attributed to the need to provide EHR access.
Why Synthetic Data is Essential for the Internet-of-Medical-Things
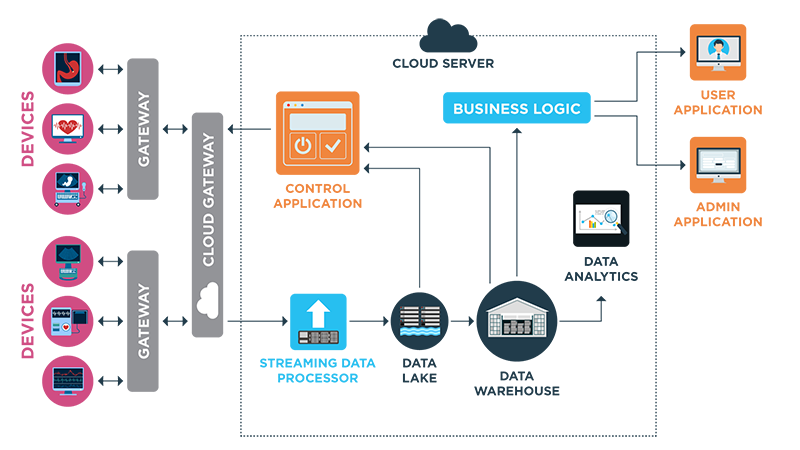
In addition to the challenge of variety and volume of data, healthcare organizations face the barriers of patient privacy and the need to protect proprietary analytical models. Synthetic data automation solves this by letting testing teams self-serve and generate their own data easily, without relying on data from the production environment.
Providers can use synthetic data to test new EHR systems before they interact with any sensitive patient data. Insurers can use it to test cost and risk models to ensure accuracy before they implement those models in real-world scenarios. Both insurers and care providers can leverage synthetic data automation to test analytics and AI tools on robust, purpose-designed data sets without accessing proprietary or patient information.
As a result, synthetic test data for healthcare will help fuel the modernization of informatics, analytics and EHR software because it addresses both the lack of interoperability in health systems and the need for ever-growing volume and variety of data as these systems scale with an organization’s needs and patient-centric models of care.
The only true limitations to synthetic data are how much data can be created and the types of data that can be generated in a short amount of time. There are also some core capabilities that are especially beneficial for the healthcare industry, which GenRocket has built into its platform:
- HIPAA Compliant, since GenRocket does not interact with production data
- Support for healthcare data exchange standards like X12 EDI and FHIR
- Ability to rapidly create a large variety and volume of data to simulate information flow from internet-of-medical-things devices
- Built-in analytics and reporting for administrators
- Data migration capabilities for leveraging synthetic and production data together as needed
With synthetic test data automation, developers and QA teams can streamline their modernization efforts and drastically expand their test coverage. With GenRocket, healthcare testing and QA teams can generate synthetic data fit to any healthcare standard on demand, including FHIR and X12 EDI.